Hearing loss affects nearly half a billion people, but only 17% use hearing aids. Imagine if only one person out of every six people needing corrective lenses had them. The consequences of untreated hearing loss cost the global economy an estimated $750B annually, due to factors such as stigma, cost, and poor performance in social situations. The low adoption rate exacerbates the challenges in making treatment accessible and affordable to all.
Femtosense’s AI technology aims to make hearing aids more accessible with better performance, compact form factor, and cost suitable for widespread use. The company’s Sparse Processing Unit and speech algorithms provide 100x more power efficiency and 10x more memory efficiency than competitor solutions, making Femtosense a leading choice for next-gen hearing aids.
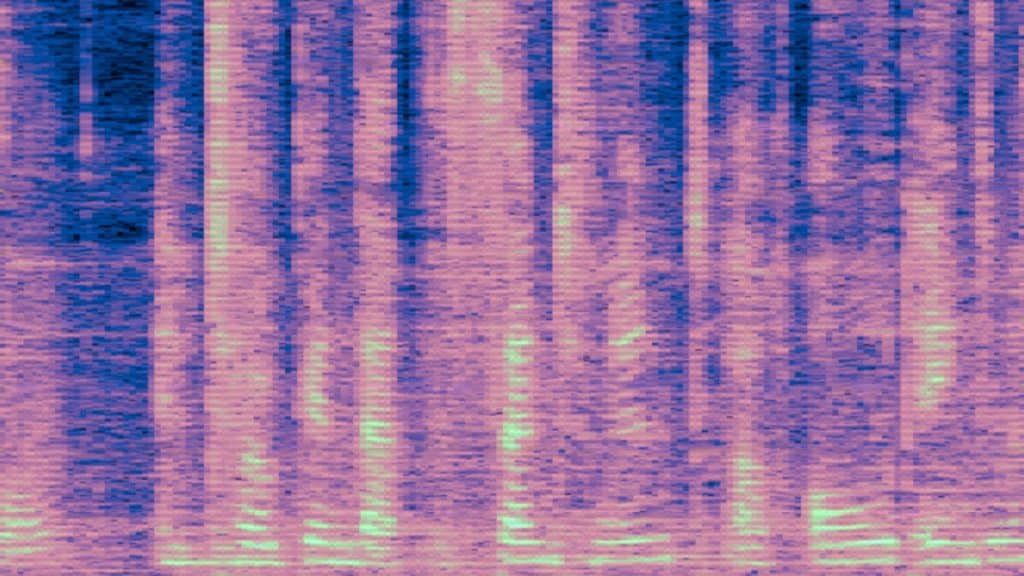
The Silent Majority
The stigma associated with wearing a hearing aid can prevent people from seeking the help they need, leading to delayed treatment and potential communication difficulties. Small form factor hearing aids, namely in-the-canal (ITC) and completely-in-canal (CIC) models offer a discreet solution, but they may not have the performance and features of larger behind-the-ear models. Attaining high performance, a small form factor, and a rich feature set is an elusive goal for manufacturers. Additionally, the cost of hearing aids can also be a barrier to access, especially for people with mild to moderate hearing loss. This can lead to the use of cheaper personal sound amplification devices that lack the quality of premium hearing aids.
Setting cost and stigma aside, many people with hearing loss, as well as those with normal hearing and neurocognitive disorders, advanced age, and traumatic brain injury, report difficulty in understanding speech in noisy environments. Despite the use of classical signal processing in premium digital hearing aids, a large number of hearing-aid users remain dissatisfied with their performance.
The Status Quo
Today’s digital hearing aids enhance speech clarity in noisy environments through: (1) a classical method that isolates and enhances frequency bands related to human speech from the environments, and (2) a beam-forming approach using on-board microphones that concentrate on the area in front of the listener. While these two commonly-used techniques have attempted to mitigate the speech-in-noise problem, many hearing aid users report that their hearing aids do not filter enough noise, degrade speech naturalness, or don’t perform well with non-stationary speakers.
Femtosense has decided to bring innovative technology to overcome these limitations.
Going Deep
Deep learning algorithms differ from classical techniques as they aim to learn what speech is and what is not, instead of relying on manual filters that identify speech frequencies. They perform better in noisy and challenging environments like restaurants, airports, and busy areas because they have been trained on a wide range of noises and environments. Additionally, deep learning algorithms reduce sudden loud noises that hearing aid users may find uncomfortable, such as breaking glass or silverware clanging.
Deep learning begins with a dataset of clean speech and speech with added noise. The audio is transformed into a spectrogram matched to human hearing; a time-varying filter is predicted for each frame to remove the noise. The filtered spectrogram is then returned as sound and played through the hearing aid speaker for clearer speech.
Deep learning algorithm adjusts its parameters by comparing the actual clean speech to the speech cleaned by the network and minimizing the difference, measured by metrics like SNR and speech quality. Training happens on specialized GPUs and once complete, the network can remove noise in real-time on another processor.
Research and Reality
Deep learning algorithms have achieved good results in experiments and research, but there are challenges in deploying them in hearing aids. One challenge is the intense computation required, which has only recently become feasible with advances in processors like CPUs and embedded GPUs. To minimize computation for deep learning algorithms, the neural network size must be decreased, resulting in fewer calculations per audio frame. There are two approaches to achieve this:
- Quantization-Aware-Training (QAT) reduces network weight and activation precision to decrease model size and computation but quantization error must be simulated and calibrated to prevent performance drop.
- Sparsity-Aware-Training (SAT) uses compression techniques to simplify the model and improve performance, by pruning with a larger model and gradually removing unnecessary connections during training.
Limited progress in sparsity research is partly caused by hardware that is not optimized for sparse workloads, leading to limited benefits and a lack of motivation to continue research. However, there is a growing interest in applying the theoretical benefits from research to real-world applications. Femtosense is dedicated to addressing this ambitious goal through both hardware and software fronts, with a goal to achieve real-time speech enhancement for hearing aids that balances noise reduction, speech quality, and energy efficiency.
The SWAP Balancing Act
The computational power problem in hearing aids faces trade-offs defined by “SWAP-C” constraints (Size, Weight, Area, Power, and Cost). The limited space for processors in hearing aids, particularly CIC and ITC form factors, exacerbates these trade-offs. Larger chips with more transistors or cores tend to have more processing capability, but also consume more power. Enhancing neural networks often involves increasing model size, which affects chip size, memory, and cost. These factors interrelate, making it difficult to balance size, power, memory, processing capability, and cost in a single solution.
Latency is a critical constraint to be considered in speech enhancement, as it affects the processing time for audio frames using a neural network. Excessive latency can negatively impact users such as hearing their own voice and cause poorer quality, causing “tin-like” or robotic speech. The accepted limit for total end-to-end audio path latency by many hearing aid engineers is 10 ms, but lower is often desired. Higher clock rate may appear to solve latency problems, but it increases power consumption. Running algorithms on batteries severely limits the processors and neural networks that can be used, operational speeds, and heat dissipation around the ear.
To be suitable for a hearing aid, we require a compact and efficient processor that can fit inside the device, enhance speech intelligibility without overheating, consume minimal power to last all day, and achieve all of this within acceptable product margins, to produce affordable hearing aids that are accessible to those who need them globally.
Hear Here for Femtosense!
Femtosense has tackled the challenging problem of enabling speech enhancement for hearing aids with the fully integrated solution; this hardware-algorithm problem is solved simultaneously by our Femtosense Sparse Processing Unit (SPU) and proprietary speech enhancement network.
Our SPU co-processor hardware features a customized speech enhancement neural network with high sparsity levels to reduce neural network size and power consumption, approximately by 10x smaller and 100x more efficient compared to today’s microprocessors and state of the art networks respectively. It provides superior best-in-class performance, in objective intelligibility measures such as PESQ and STOI, as well as in SNR improvement.
The SPU runs our proprietary algorithm within strict latency requirements for hearing aids, consuming less than 1mW at 8ms latency and running for hours on battery power. At 3mm2, the SPU efficiently fits behind-the-ear and in-canal form factors, utilizing silicon real-estate more efficiently than non-sparsity solutions
We offer optimization tools for implementing sparsity and quantization. Our hardware can simulate algorithm performance (power and latency) after training and optimization, which simplifies the iteration process for developers to quickly determine hardware and network compatibility. We also provide assistance with deploying your algorithm to the SPU in just a few lines of code.
To summarize, Femtosense offers the only fully integrated speech enhancement solution for premium digital hearing aids. The SPU is a cost-effective and high-performing solution to the speech-in-noise problems affecting millions of hearing-impaired individuals. By integrating into hearing aid SoCs, manufacturers can lead the market with this feature. Femtosense is eager to collaborate and improve the lives of people worldwide with the future of digital hearing aids.
Learn More
Want to hear the solution live in action? Want to read more about our proprietary algorithm? Want to get hardware specifications, order an eval kit, or reserve test chips? Reach out to us at [email protected] to schedule an in-person demo, request documentation, or chat about other applications like keyword detection, sound and scene ID, neural beamforming, or biosignal classification.
Femtosense Inc.
The future of AI is sparse.